Share
As the Centre’s 2020 Predictive Analytics Fellow, I conducted research in June and July to better understand the current processes for implementing predictive analytics in humanitarian action. In this blog, I present my findings and recommendations for improving the use of predictive analytics in support of anticipatory action. I hope the outcomes of my research can inform the global community of practitioners working to act before a crisis intensifies.
The focus of my research was based on consultations with the Early Action Focus Task Force, a group of stakeholders working to ensure coherence in anticipatory action in the sector. I aimed to answer the following questions:
- What are the emerging best practices for model development and use?
- What pitfalls do organizations face in using predictive models for anticipatory action?
- How can we improve the implementation of predictive analytics in humanitarian settings?
My research consisted of a literature review, key informant interviews, and the development of case studies. In conducting the literature review, I focused on technical academic papers, humanitarian project documents, and independent reviews of the application of predictive analytics within the development and humanitarian sectors. For the key informant interviews, I spoke with ten experts working on predictive analytics from four humanitarian organizations: the International Federation of the Red Cross and Red Crescent Societies (IFRC), the Start Network, the UN Food and Agricultural Organization (FAO), and OCHA. For the case studies, I focused on FAO-led pilots in Colombia and Sudan and an OCHA-led pilot in Bangladesh, with an emphasis on documenting the process that these organizations followed to apply predictive models.
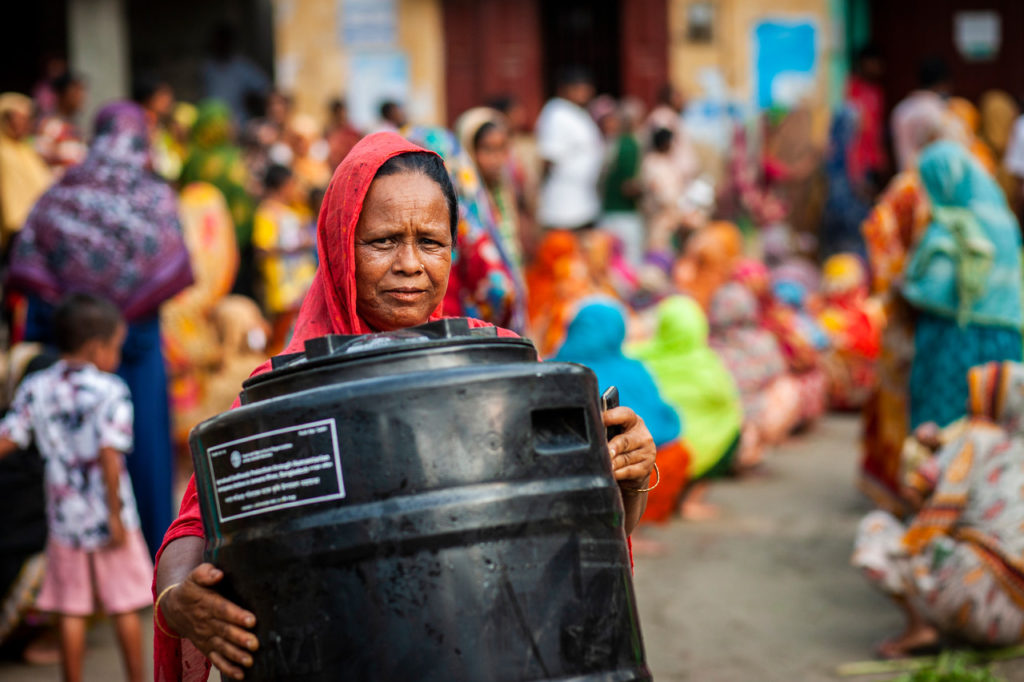
The key findings from my research are as follows:
- Many organizations are not clear on the questions driving modeling work or how predictive analytics fits into how their overall analysis efforts.
- Many organizations have insufficient expertise related to the technical development, contextual adaptation, and programmatic use of models. This may lead to inefficient or inaccurate model development and could also present ethical concerns.
- Local participation is essential in the design and ownership of predictive models, but is often overlooked or underdeveloped.
- Organizations often neglect or fail to properly implement technical review phases. In some cases, this has led to the implementation of inaccurate models.
- Significant challenges persist in relation to data gaps and data quality, limiting the viability and accuracy of model development.
Based on these findings, I offer the following six recommendations for improving the implementation of predictive models in support of anticipatory action:
- Invest in filling data gaps and ensure that quality data is used for modeling. Accurate data is key to rigorous model development. Predictive models built on incomplete or inaccurate data are of little use. While proxy information such as remote sensing can be helpful in some circumstances, there is a need to invest in primary data gathering to further enhance a model’s accuracy.
- Involve technical partners, local stakeholders and implementing organizations in the development of triggers and related disaster risk financing frameworks. This can improve accuracy of models and foster buy-in for models by local governments and other stakeholders.
- Build and retain internal technical capacity for model development and use. Internal technical capacity should complement working with technical partners and is beneficial to accurately develop models, but also to ensure oversight throughout the program life-cycle.
- Use predictive models in conjunction with traditional methods of analysis and evaluation. While predictive models can highlight the onset of a shock, they should be used alongside other methods such as needs assessments and community perception surveys.
- Ensure that trigger mechanisms are linked to financial plans. The development of standard operating procedures (SOPs) regarding the financial response to a trigger will help avoid delays once a trigger is activated.
- Engage in multi stakeholder peer review processes with technical and local partners. This can help ensure the modeling is both technically sound and also reflective of the local context.
If you are interested in learning more about this research, download my final report which includes more details from the key informant interviews and case studies:
The Centre’s Predictive Analytics team is available to support this community through peer review of partner models and validation of candidate models for anticipatory action frameworks. Learn more about this work here.