Share
Since opening in 2017, the Centre has focused on increasing the use and impact of data in the humanitarian sector. Critical to this mission is their management of the Humanitarian Data Exchange (HDX), an open platform for sharing data across crises and organizations. The HDX roadmap for the coming years includes evolving the platform to be more sophisticated in its processing of data at scale, and preferencing data quality over data quantity.
With this in mind, the HDX team asked us to research and prototype possible quality measures for humanitarian datasets that are hosted on the HDX platform. We share our main findings below and hope that they are relevant not just for the Centre but the wider humanitarian community. The full report can be found here.
The Data Nutrition Project (DNP) is a non-profit that formed in 2018 to develop tools and practices to improve transparency into datasets. DNP takes inspiration from nutritional labels on food, aiming to build labels that highlight the key ingredients in a dataset such as metadata and demographic representation, as well as unique or anomalous features regarding distributions, missing data and comparisons to other datasets.
Our work with the HDX team focused on understanding their quality assessment practices, how users find and select data on HDX, and different conceptions of data quality in the humanitarian sector. Using two preselected datasets as examples, we prototyped a way to understand quality through a summation “score” that indicates whether the information is available, rather than a normative grade on the information itself. We also explored whether this effort could be undertaken by third parties or automated. The main tension for data quality measurement on HDX is between usefulness and scalability; in our experience, this is the most common challenge in dataset transparency efforts.
How HDX Assesses Data Quality
The purpose of HDX is to make humanitarian data easy to find and use for analysis. Data is shared through approved organizations and all new and updated datasets are reviewed by the HDX team. Data quality is measured against a set of criteria that includes first and foremost the data’s relevance to the humanitarian community. Other aspects include the data’s accuracy, timeliness, accessibility, interpretability and comparability, as first described when the platform launched in 2014 and later documented in a quality assurance process. Aligning the data pipeline to quality principles and responsible use highlights the critical relationship between a data contributor and the HDX team for the assessment and communication of data quality.
We read and analyzed approximately a dozen reports about data quality in the humanitarian sector in order to compare criteria across several organizations. As a point of departure, we used the Generic Data Quality Assurance Framework (UN, 2015) as a baseline (see chart below). Notably, we saw a gap in the literature published by HDX around how “credibility” is defined and assessed. In particular, the extension of credibility from the organization (we find this organization credible) to the data itself (thus the data is also credible). We added this element into the matrix (in light blue, below), and included this principle in our research into data quality on HDX.
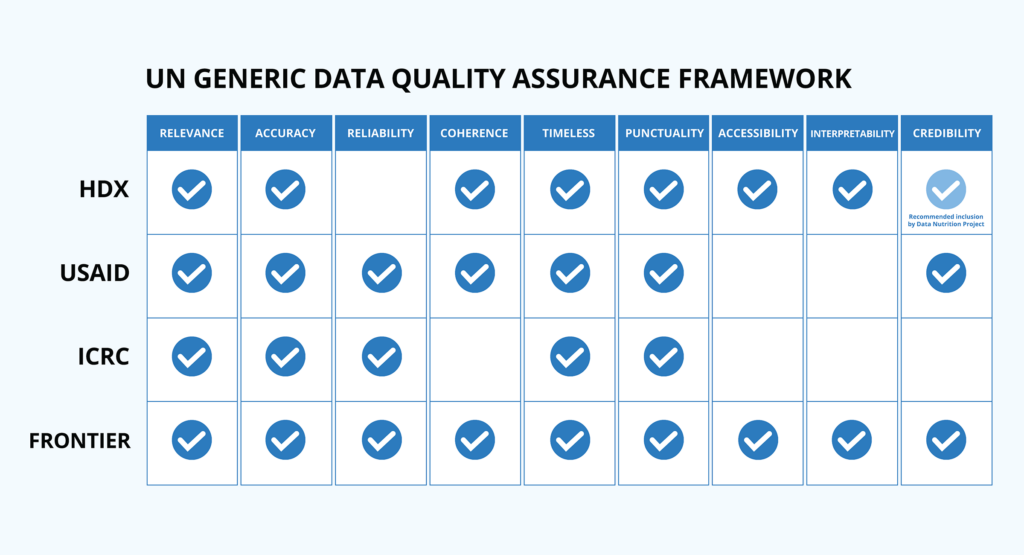
Data Quality Measurement Challenges
There are many challenges that can be impediments to data quality. This is certainly the case in the humanitarian sector, where crises unfold quickly and data capture will almost always be imperfect, often as a consequence of the need for rapid collection. These challenges emerge as a series of tradeoffs or tensions that require finding a balance, especially when it comes to identifying which quality measures to capture, and how to present them to an audience.
Challenge 1: Identifying scoring methods that are succinct while not overly simplistic
It is important to identify quality metrics that are easy to understand and compare. However, simple numbers such as quality scores or grades can risk being reductive and hard to parse. A single score to compare across inconsistent data types or domains may not only be useless but can also seem arbitrary. However, quantitative measures can be easier to automate and thus easier to scale.
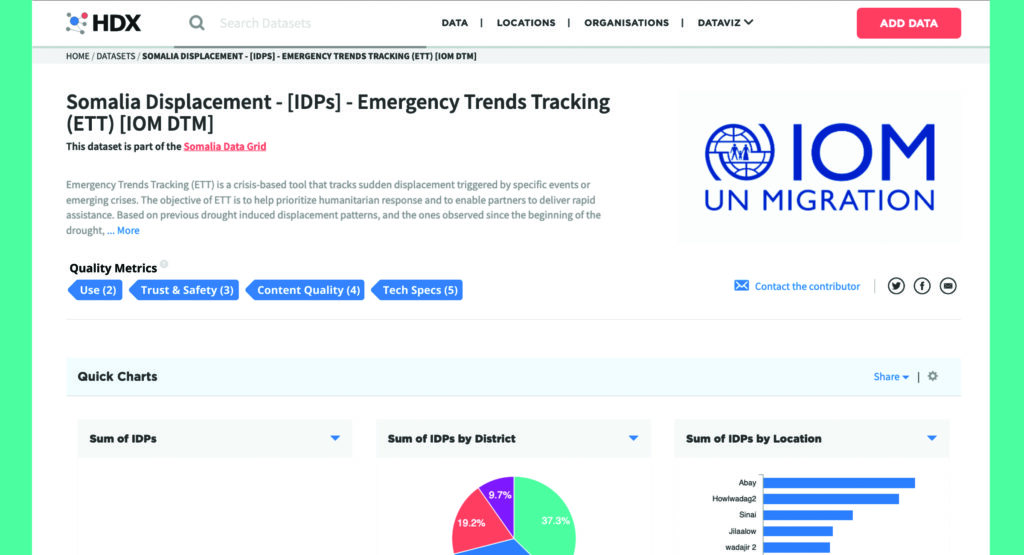
Challenge 2: Balancing scalable (quantitative) & comprehensive (qualitative) measures
Qualitative information helps mitigate some of the concerns above, as it is often more context-aware than quantitative statistics alone. However, qualitative information is also resource-intensive to collect and often is domain-specific.
Challenge 3: Communicating quality to motivate rather than disincentivize
The hope is that quality measures will facilitate better data choices and motivate the publishing of higher quality data by changing user expectations and data collection habits. However, depending on how measures are disclosed and how scores are determined, it is also possible that certain measures could discourage full transparency when sharing data or even the sharing of data at all.
Challenge 4: Building a quality framework that balances flexibility with consistency
As the humanitarian sector changes over time with respect to crises and data needs, any discrete quality metrics will also likely change. For these reasons, whatever is built will need to be adaptable. However, consistency is also important, so that datasets from different time periods can be compared, and so that dataset owners and site visitors can develop familiarity and comfort with the site.
Challenge 5: Prioritizing (but not overburdening) subject matter experts
Domain knowledge is essential for accurately interpreting or validating data, especially in highly specialized sectors with rich information (such as definitions, vocabulary, approaches). Most organizations, especially those in the humanitarian sector, have limited resources with respect to time and personnel for validating datasets. It is important to determine how responsibility for contextualizing datasets gets distributed along the data pipeline, without overburdening subject matter experts or creating bottlenecks to publishing the data.
Our Findings
The research we conducted with the HDX team and stakeholders gave rise to a number of key findings, which informed our recommendations and design proposals.
Finding 1: HDX is best positioned to define rather than assess quality
HDX is best positioned to: (1) define the framework for the quality of datasets on HDX; (2) facilitate the gathering of this information from data organizations; and (3) provide a display of this information to data users on HDX, designed in alignment with user needs. It is our view that HDX is not well positioned to create or uncover this information themselves, as there is tremendous domain knowledge required to do this for all datasets across all geographies.
Finding 2: There is an opportunity to leverage existing quality measures
Data quality assessment is already conducted on HDX, at different times and displayed in disparate regions of the site. This provides an opportunity, as a first step, to aggregate, prioritize and organize information that has already been gathered, such as whether disclosure control has been undertaken or if a dataset includes place-codes. Further ambitions to gather more information on data quality should come after bringing transparency to the valuable information that HDX already has.
Finding 3: Domain experts and third-party validators can provide complementary value
Many data organizations have quality frameworks or assessments for their own data, and these could be included to communicate known issues or certain strengths of a dataset within its domain. Working in collaboration with other organizations can lend institutional validation to these quality frameworks and can provide needed domain expertise. Using these external third-party-determined metrics also encourages other organizations to consider adapting their use frameworks to include quality, which can drive cultural change around responsible data usage.
Finding 4: Automation of quality assessment tasks can enable scale
Automating certain tasks will enable quality measures to be assessed and applied at scale. For example, pre-filling or requiring specific metadata fields can make it easier to enforce and collect quality information about datasets. HDX could also consider requiring the submission of metadata around intended usage or other domain-specific or format-specific information. This ultimately enables comparison across and within geographies, which is critical for supporting dataset selection on HDX.
Finding 5: Primary use case for quality measurement is data selection
The primary reason for having quality measures is to improve dataset selection, with additional use cases including dataset comparison or dataset combination. The availability of legible, digestible measures, such as format, update frequency, and uses and restrictions, permits users to quickly scan for their particular needs.
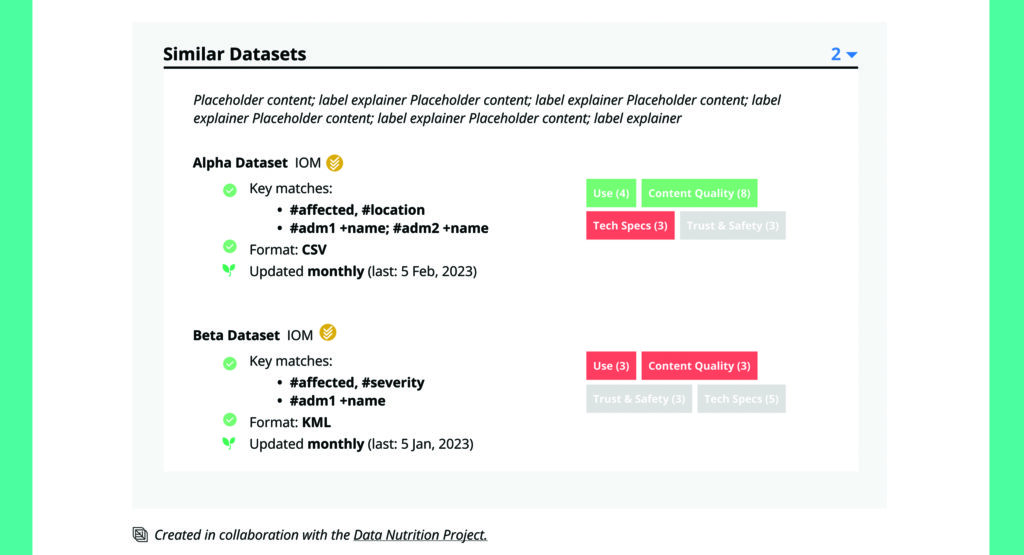
Next Steps
Based on our collaborative research, there are several possible avenues for implementation on HDX. The team is already experimenting with comparing sources of data to help users with dataset selection. There may be opportunities for the automation or use of algorithms to identify quality, which is something the team is exploring as part of the HDX roadmap. However, technology can also oversimplify, introduce bias or obfuscate complexity and should be used to support rather than replace human decision making.
Many fields that involve data-driven decision making are only now starting to ask questions about dataset quality – questions that HDX has already answered and started to build into its systems. With a concrete, phased approach, HDX can implement quality measures for data in a way that meets its users’ needs and sets an example for many other fields. DNP looks forward to continued collaboration in this process. We welcome comments or feedback at info@datanutrition.org. Watch our webinar on data quality with the HDX team here.