Share
The Centre spoke with Dr. Muhammad Imran, Scientist and Lead of the Crisis Computing team at the Qatar Computing Research Institute (QCRI), which is part of the Hamad Bin Khalifa University (HBKU). His team is dedicated to bringing artificial intelligence and machine learning techniques to humanitarian action. We discussed AIDR, the group’s publicly available AI-based platform for disaster response, the role of social media in understanding crises, and what’s next for predictive models in the sector.
This interview was conducted by Elizabeth Wood, the Centre’s Communications Manager. It has been edited and condensed for clarity.
Tell us about your work with QCRI.
I lead the Crisis Computing team at QCRI-HBKU based in Doha, Qatar. After joining QCRI as an intern in 2012, we published several papers and a proof-of-concept system to collect and classify Twitter messages during disasters. As I came to understand the humanitarian domain, I found several unmet needs. Most importantly, I realized that artificial intelligence has tremendous applications to solve real-world humanitarian challenges. It can help predict an outbreak or epidemic, analyze millions of images to find evidence of damage, or find reports of missing people. AI-powered drones can survey large disaster areas for early damage assessment. There has been a start to leveraging AI in this sector, but I believe it’s just the beginning.
How does the AIDR platform work?
Information scarcity leads to delays in humanitarian response. The main focus of the Artificial Intelligence Disaster Response (AIDR) platform is to find information through social media before other sources may be available. AIDR uses text and images from platforms such as Twitter to fill information gaps and provide real-time, processed data. Then, we look for humanitarian information from this data. We’ve tried to make AIDR a flexible and customizable system so that anybody can create and train a machine learning model to address their specific needs.
What are the benefits and drawbacks of using social media data for insight?
Social media contains some of the most actionable information in the early hours after a disaster. Yet, it also poses more challenges than other data sources like sensors, satellites, and drones. On social media, people often post personal information, opinions, sentiments, prayers, and thoughts that are related to a crisis, but are not useful for humanitarian purposes. They also use shortened words, slang, different languages, and other contextual information, which means our machine learning techniques have to be very robust. In this way, social media can feel like a big, giant haystack that builds up as minutes or even seconds pass. The challenge is to extract the needle in that giant haystack.
Do you have examples of using social media data to support a specific crisis?
When Hurricane Dorian began forming in the Atlantic Ocean on 30 August 2019, we activated the AIDR platform to find relevant images on Twitter. By the time it made its first landfall on 1 September, we were already capturing images showing damage. We soon coordinated with volunteers from the Montgomery County emergency response team to perform early damage assessment with these images. This usually involves a lot of manual steps: searching for images, viewing and downloading them, authenticating the users posting them, and so on. We automated these processes to only share severe damage. Ultimately, we collected approximately 300,000 images and only around 13,000 showed any damage—an information overload reduction of over 95 percent.
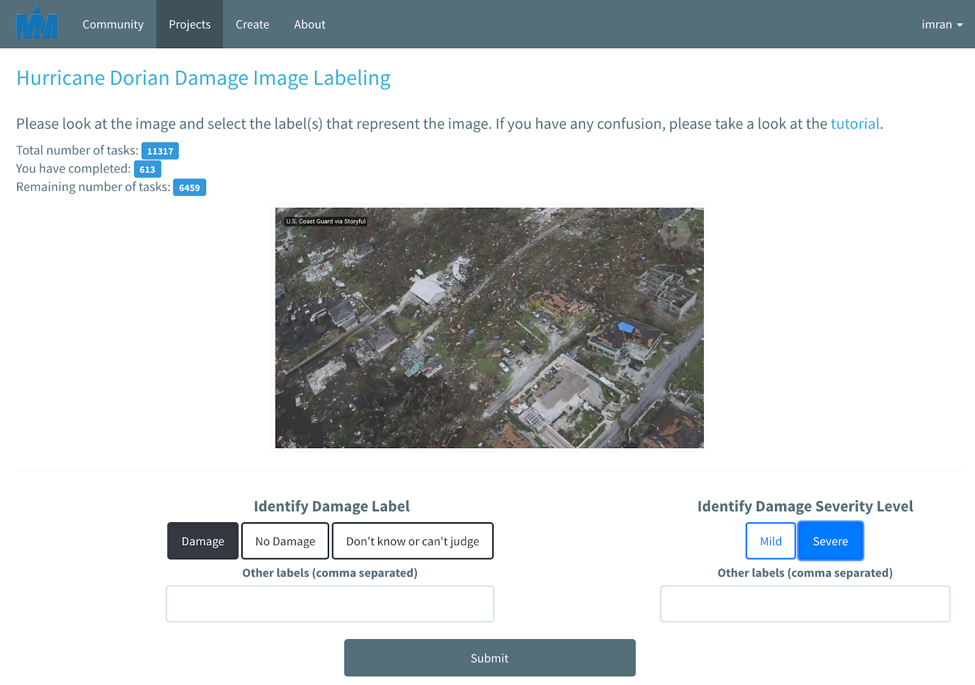
How does your team work with the Centre?
We have an Memorandum of Understanding between the Centre for Humanitarian Data and QCRI. We have been working with the team to use AIDR to identify Twitter posts related to attacks on education in Africa and the Middle East. QCRI also shares the analysis of Twitter data through HDX so that we can reach a wider community. Our goal is to address direct information needs, offer complementary signals, help in triangulation, and so forth.
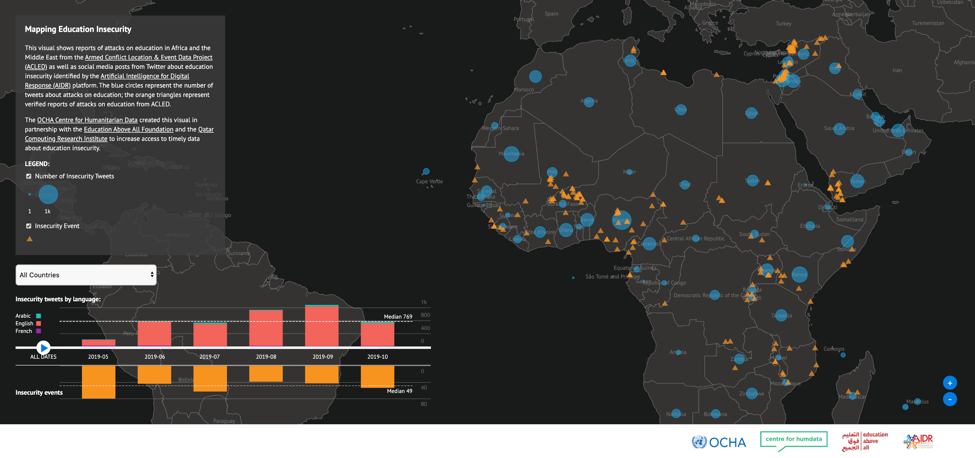
What are the primary challenges your team faces working in this sector?
One challenge is the growing gap between technologies and stakeholders. No technology will be used if its not aligned with stakeholder needs. On the other hand, technologists cannot develop new systems if they’re not aware of how to help. To bridge this gap, I would say more workshops, webinars, or such events need to be conducted on both ends—both technological and humanitarian—to help each group synchronize and fully understand each other.
It’s fascinating to see how much the community has grown since we began in 2013. More AI researchers are taking an interest in solving humanitarian challenges, but even more would be welcome. AI techniques rely on large amounts of data to make inferences, predictions, or decisions. With recent advances in deep neural networks, researchers are achieving breakthroughs in many different domains, including health informatics and machine translation. However, there is a lack of standard datasets and benchmark results on which new models can be compared and evaluated against in this sector. When you set a benchmark for a dataset, there has to be a presence of different regions, different languages, etc. to build standardized datasets and to reduce potential bias.
How do you see the role of predictive modeling in disaster response?
Predictive modeling, data mining, and machine learning techniques can help analyze large amounts of historical data and be able to make predictions about unanticipated future disaster events or crises. The goal is to find patterns to identify future risk. We have been collecting data since 2013 from all past major disasters. We’re trying to learn from these datasets in order to create models that can actually help us predict a future disaster.
As with Hurricane Dorian, our real-time imaging processing pipeline of posts from Twitter represent four tasks in a predictive model. The first is to collect images from social media. The second is to remove duplicates and delete them. People tend to share the same image again and again, so we have machine learning models that can identify and eliminate duplicate images. The third deals with image relevancy, meaning the image does not show cartoons, banners, advertisements, or explicit content. If the image is relevant, then the fourth and last task is the damage assessment. This identifies whether the image shows damage or not, and if so, the severity of the damage.
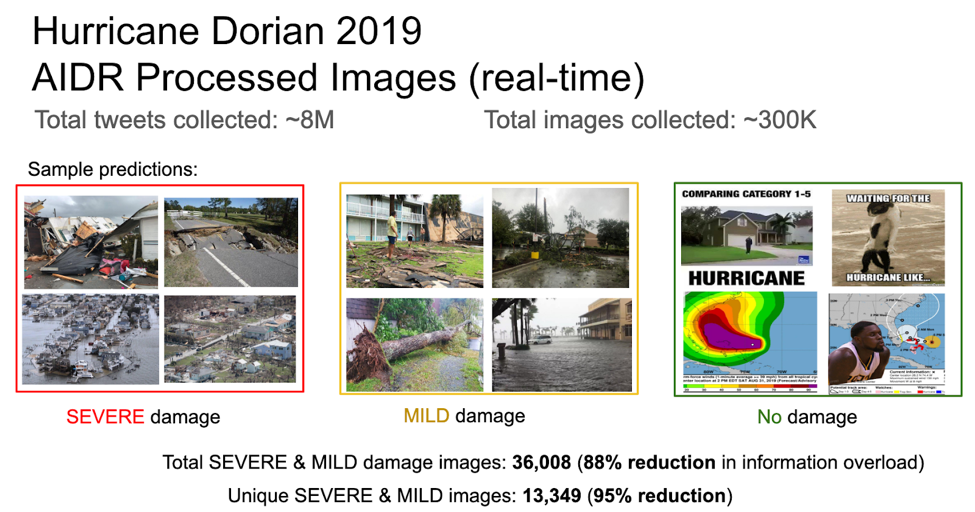
How can people get involved with your team and start using AIDR?
AIDR is a public service. Anybody can go to the website and start collecting textual data for humanitarian purposes. If people need help, we have documentation available. Image processing is limited to humanitarian organizations because it is a resource-intensive task. Humanitarian organizations can put in a request and it will be available to them free-of-cost during a disaster.
What’s next for AIDR and QCRI’s Crisis Computing team?
Right now, our models assess the damage from an overall image, but in a given image, there could be different objects, such as trees, houses, bridges, and so on. We’re working on identifying the object-level of the damage. In addition, research in this domain so far has mainly focused on situational awareness aspects, as in trying to understand a disaster as it unfolds. But a big gap remains. For example, if someone is trapped on street X and has need Y, we should be able to capture that immediately and give it to humanitarian organizations so they can get relief to that individual. Currently, only one percent of Twitter data is geo-tagged. For the remaining 99 percent, we have to rely on other techniques to infer where the message is coming from.
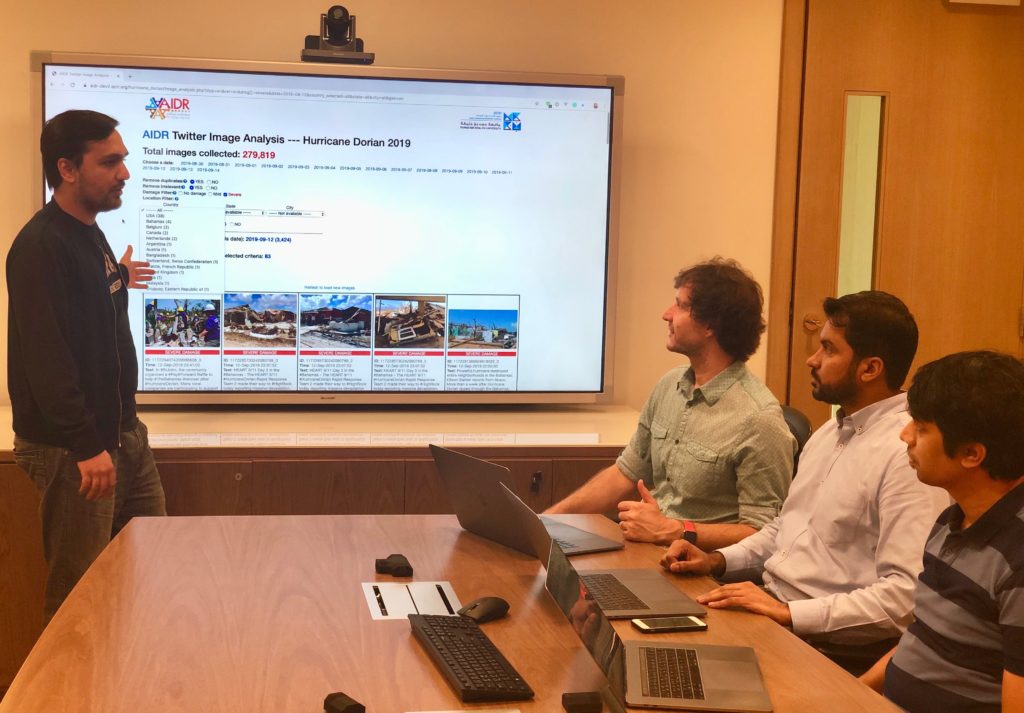
What do you love about what you do?
That’s my favorite question. First, I would start with the fact that our work can help save lives and alleviate suffering by understanding the urgent needs of affected populations. Second is the commitment of our team members. We all share equal passion for working with humanitarian organizations and providing the data assistance they need. Third, I appreciate how Qatar has invested in the humanitarian and education sectors by creating a world-class institute focused on computing. Here, we have the freedom to pursue research and develop state-of-the-art technologies for humanitarian purposes.